Imprecise Probabilities in Quantum Mechanics
Posted on April 26, 2022 by Alessio Benavoli (edited by Ignacio Montes)[ go back to blog ]
The beginning
I was glad when Ignacio Montes, SIPTA’s Executive Editor at the time, invited me to write a post on my research journey on Quantum Mechanics (QM), a journey undertaken together with Alessandro Facchini and Marco Zaffalon.
Everything started with an article by Hans Christian von Baeyer, titled Quantum Weirdness? It’s All in Your Mind, published in Scientific American 2013. The article is about Quantum Bayesianism (QBism), a model of QM in which the wave function exists only as a mathematical tool employed by an agent to assign his or her personal belief that a quantum system will have a particular property.
QBism is part of a broader research effort to reconstruct the quantum formalism from simple principles (subjective probability, information theory,…), which started with an article by Lucien Hardy in 2001.
Putting desirability in the equation
Going back to von Baeyer’s article and QBism, our question was
“can we derive the main axioms of QM using the gambling (desirability) foundation of probability theory (à la de Finetti or Williams-Walley)?”
What are the gambles in this context? We wanted to do that because the method used in QBism to connect QM to the foundation of subjective probability is not well formalised (that is, by deriving QM from coherence rules in a betting system).
In our first approach [benavoli16], we took the matrix formulation of QM, in which the observables (things one can measure with an experiment) are described by Hermitian matrices and used these matrices to define gambles on the results of a quantum experiment. The space of Hermitian matrices is a vector space and, in this context, positive semidefinite Hermitian matrices play the role of nonnegative gambles, and negative definite Hermitian matrices play the role of negative gambles. We defined the standard axioms of (almost or strict) desirability in this vector space:
- accept nonnegative gambles;
- avoid negative gambles;
- additivity, continuity, and positive scaling.
When we compute the dual of such a coherent set of desirable (matrix) gambles. The result is a closed-convex set of trace-one positive semidefinite Hermitian matrices. These matrices are called “density matrices” in QM.
Surprisingly, desirability axioms were everything we needed to prove the first postulate of QM which states:
“Associated to any isolated physical system is a complex vector space with inner product (that is, the space of Hermitian matrices) known as the state space of the system. The system is completely described by its density matrix, which is a positive semidefinite matrix with trace one, acting on the state space of the system.”
We also proved the other main postulates of QM using probabilistic concepts like updating, marginalisation and independence.
Unfortunately, the approach based on matrix gambles did not allow us to understand why QM radically deviates from classical predictions. QM is a consistent (rational) theory, this is what we proved using desirability, but it includes phenomena like entanglement which appear weird to us (that is, we cannot explain entanglement using our common sense (classical physics)).
Adding bounded rationality
Luckily, in the same period, we were working on an apparently unrelated topic called bounded rationality, or more precisely, algorithmic rationality [benavoli17]. The goal here is to understand how an agent can make rational decisions using limited computational resources. For instance, a basic principle of rationality is that a rational agent should always accept a nonnegative gamble, because it is always favourable. However, when the space of possibility is infinite, a gamble is a function. Therefore, evaluating the positivity of a gamble can be very hard computationally (if not impossible).
In this case, we can relax the requirements of rationality to account for the limited computational resources of the agent. For instance, we can require that the agent should only accept nonnegative gambles if their nonnegativity can be evaluated efficiently (that is, in polynomial time).
We proposed an instance of an algorithmic rationality theory working with gambles which are polynomial functions. Evaluating the nonnegativity of a polynomial is in general NP-hard and, therefore, we relaxed the notion of nonnegativity by considering a subset of the nonnegative polynomials, that is the subcone of the sum-of-square (SOS) polynomials. These are polynomials which are a sum of squares of polynomials and, therefore, nonnegative. Determining if a polynomial is SOS is equivalent to checking the feasibility of a semidefinite programming problem, which can be solved in polynomial time. Therefore, an agent can evaluate the membership of a polynomial to the SOS set efficiently. We then defined the following notion of algorithmic rationality (desirability):
- accept SOS gambles;
- avoid gambles whose negation is SOS, which are strictly negative;
- additivity, continuity, and positive scaling.
We then computed the dual of an algorithmic coherent set of desirable gambles, which resulted in a closed-convex set of matrices. They are a sort of moment matrices (similar to covariance matrices).
Intriguingly, the above duality proof is exactly the same proof we used to derive density matrices in QM. This allowed us to make a connection between algorithmic rationality and QM or, equivalently, to answer the following fundamental question: why does QM has the form it does? Can it be that QM is simply a theory of algorithmic rationality?
So, we went back to our QM paper, rewrote the matrix gambles using polynomials (Hermitian matrices go together with quadratic forms, which are polynomials) and showed that we can derive QM from two principles: coherence and computation [benavoli19].
In this new framework, we can easily understand “quantum weirdness”: it is just a clash between the standard definition of coherence/rationality we use in classical probability (and so in classical physics) and algorithmic rationality.
Entanglement is a Dutch book from the point of view of a fully rational agent. Since the cone of negative gambles in QM is smaller than the cone of negative polynomial gambles, a quantum agent can accept a negative gamble without being irrational. From the perspective of a fully rational agent, the quantum agent incurs a sure loss (although the quantum agent is indeed algorithmically rational).
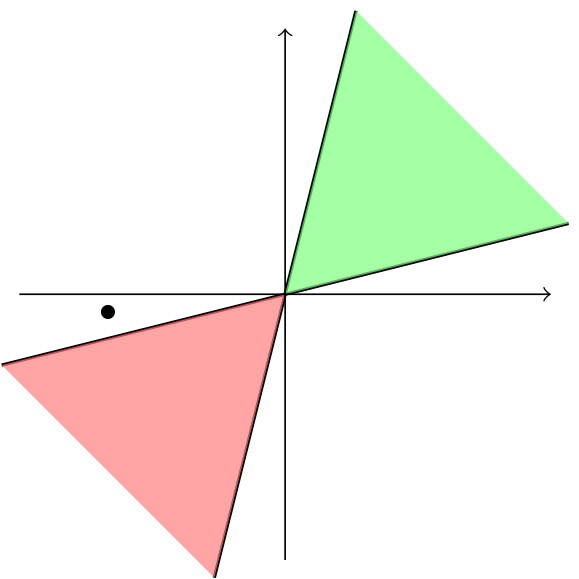
The amazing thing is that this is a property of the Universe where we live and so when we evaluate that negative polynomial gamble (through an experiment) the reward can be positive. This is what we call entanglement and it is a consequence of algorithmic rationality.
Our last contribution: adding exchangeability
More recently [benavoli21], we derived another axiom of QM, which deals with indistinguishable particles and “defines” the two types of fundamental particles Bosons and Fermions. We made this axiom to follow by coherence, computation and exchangeability (which is another fundamental concept in probability).
Where is physics in quantum physics? Apparently nowhere or, maybe, just in the computational postulate!
Main references
[benavoli16] Alessio Benavoli, Alessandro Facchini and Marco Zaffalon. “Quantum mechanics: The Bayesian theory generalized to the space of Hermitian matrices”. Physics Review A, 94:012106, 2016.
[benavoli17] Alessio Benavoli, Alessandro Facchini, Dario Piga and Marco Zaffalon. “SOS for bounded rationality”. In: Proceedings of the International Symposium on Imprecise Probability: Theories and Applications (ISIPTA 2017). PMLR 62: 25-36, 2017.
[benavoli19] Alessio Benavoli, Alessandro Facchini and Marco Zaffalon. “Computational complexity and the nature of Quantum Mechanics”. ArXiv, 2019.
[benavoli21] Alessio Benavoli, Alessandro Facchini and Marco Zaffalon. “Quantum indistinguishability through exchangeable desirable gambles. In: Proceedings of the International Symposium of Imprecise Probability: Theories and Applications (ISIPTA 2021). PMLR 147: 22-31, 2021.